The U.S. Navy and the Defense Innovation Unit announce an integrated capability for improved underwater target threat detection developed in just one year.
June 17, 2024 -- The U.S. Navy relies on machine learning (ML) models to support underwater target threat detection by unmanned underwater vehicles (UUV), but, until recently, has lacked a way to monitor and quickly improve post-deployment performance to maintain the models’ operational utility at scale. Prior to this project, systems would rely on ML models that can be slow to adapt to changing conditions or enemy tactics. AI infrastructure is required to continuously monitor and improve model performance to ensure the systems remain effective. Without this monitoring and retraining capability, ML models within these mission critical systems risk producing inaccurate or unreliable results. DIU has previously worked with the Navy to prototype and field the UUVs that run these algorithms.
In 2022, the Navy partnered with the Defense Innovation Unit (DIU) to resolve this issue by leveraging commercial technology that could be integrated into the Navy’s existing systems. Using DIU’s Commercial Solutions Opening process, the team identified commercial machine learning operations (MLOps) vendors who could collectively provide a pipeline to track, modify, and redeploy ML algorithms for underwater target threat detection.
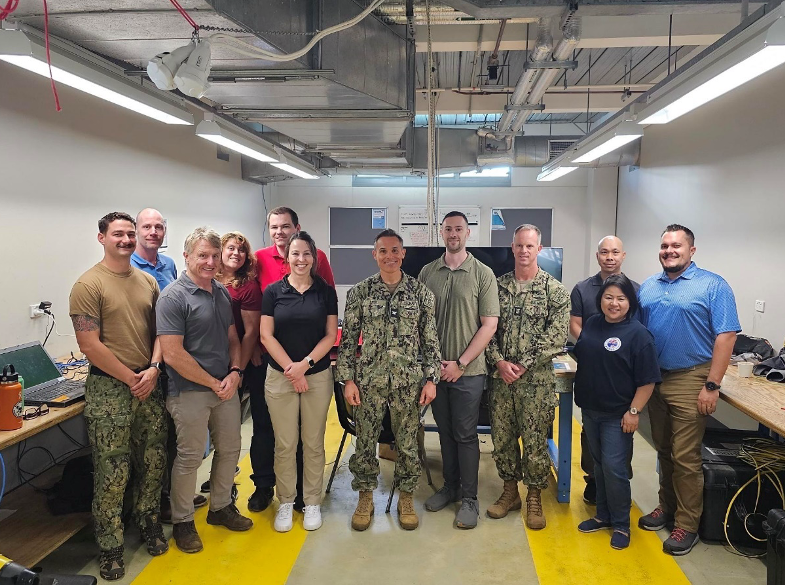
The AMMO and UGIS technology demonstration teams meet with CDRE Lieb, CTF 75.
After a competitive and thorough evaluation process, DIU awarded five prototype agreements in the fall of 2022 to Arize AI, DoAfter a competitive and thorough evaluation process, DIU awarded five prototype agreements in the fall of 2022 to Arize AI, Domino Data Lab, Fiddler AI, Latent AI, and Weights & Biases as part of Project Automatic Target Recognition using Machine Learning Operations (MLOps) for Maritime Operations (AMMO). Under Project AMMO, each vendor contributes an essential component of the overall capability. Together, these capabilities allow the Navy’s ML developers – Johns Hopkins Applied Physics Laboratory, Northrop Grumman, and SeeByte – to focus on the core tasks of model creation and refinement, allowing the tools to handle the critical aspects of deployment, monitoring, and governance.
“The partnership between the U.S. Navy and DIU has opened the pathways to commercial innovation and has produced a MLOps toolset that ensures our solutions adapt and evolve at the speed of tactical relevance, placing an enduring capability in the hands of the warfighter,” said Commander Eli Ford, Principal Assistant Program Manager, Underwater Explosive Ordnance Disposal, PMS 408 (Expeditionary Missions). “Nowhere was this more apparent than at an Integrated Battle Problem in the Fall of 2023 where sensor data was collected, new models were trained against an expanded threat environment, and updated detection models were deployed through the AMMO ecosystem back into the hands of the operator.”
The Fall 2023 exercise also revealed that the vendors’ new pipeline decreased the time needed for ML model updates from six months to a few days - a 97% decrease.
As of April 2024, each vendor successfully prototyped and deployed their portion of the broader AMMO MLOps pipeline. The Navy is in the process of awarding success memos to each vendor and transitioning this capability into production contracts, and will be expanding the technology to other naval missions. In addition, other partners across the Department are assessing how to best use the AMMO toolset to build and improve their own MLOps pipelines for other relevant use cases.
“The partnership consisting of the Navy's Project Overmatch, PMS-408 Expeditionary Missions, Test Resource Management Center, and Defense Innovation Unit resulted in a capability known as Project AMMO. AMMO greatly contributed to accomplishing our subsea/seabed warfare (SSW) mission during a trilateral Integrated Battle Problem last fall by improving our ability to detect targets of interest in a complex and dynamic undersea environment,” said Commodore Shaun Lieb, Commander, Task Force (CTF) 75.
“Working with multiple international partners, we demonstrated the ability to deploy and update our automatic target recognition models at the speed of operational relevance while simultaneously remaining confident in their performance. This embodiment of human-machine teaming gives our Sailors a powerful tool in their arsenal and increases confidence in their systems. I'm proud our warfare community could be the pathfinder and exemplar for machine learning ops, tooling, and processes that will improve many other Navy and joint service warfighting capabilities in the age of artificial intelligence,” continued Lieb.
Project AMMO sets the stage for DoD to deploy and manage ML algorithms effectively at scale. Since robust MLOps practices are crucial for ensuring the safe, reliable, and effective operation of all ML models, AMMO’s capabilities provide a foundation for building the DoD’s trust in and reliance on these new technologies, furthering the DoD’s efforts to responsibly develop and deploy artificial intelligence (AI) and ML algorithms.